Register for my upcoming Build an AI Content Team: Elevate Your Writing, Scale Your Impact course on Maven
A marketer’s guide to open-source large language models
Natalie Lambert
3/23/20243 min read
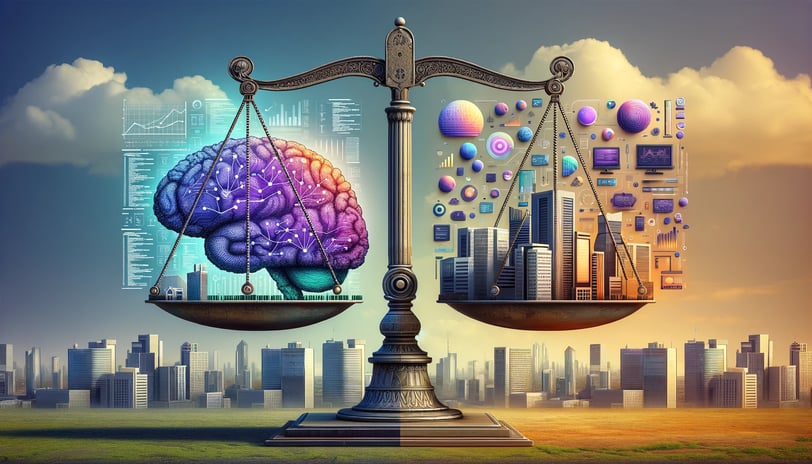
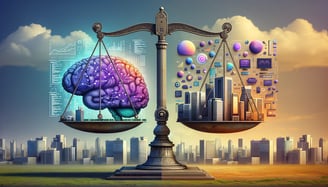
Open-source large language models (LLMs) are gaining traction and making headlines for their improved performance, lower licensing costs, and enhanced security features — key attributes for marketers. This raises the question: Should marketing teams adopt open-source LLMs over commercial solutions from companies like Anthropic, Google, and OpenAI? While open-source models offer certain benefits to marketing teams, they also present unique challenges. This post aims to provide marketers with a foundational understanding of the benefits and limitations of open-source LLMs and help determine when they might be the right choice.
Balancing the scales: Open-source LLMs in marketing
Open-source LLMs, as the name implies, are available for anyone to use, modify, and distribute without the need for licensing fees or proprietary restrictions. Examples include Meta’s LLaMA, Apple’s Ferret, and Mistral. Notable for being free to use, these models can potentially match or outperform larger proprietary models and offer deployment flexibility (either on-premises or in a private cloud) and internal management. Note: There is a range of how "open" open-source models are as noted in an article by IEEE Spectrum which comments that "Meta has made the trained model available, it is not sharing the model’s training data or the code used to train it."
This last point is important. Most commercial (or closed-source) LLMs are hosted, managed, and operated by the provider. This means organizations that want to use their data within an LLM must send that data to the provider’s cloud and storage, which often violates privacy regulations and security policies. With open-source LLMs most often being deployed on-premises or in a private cloud, organizations can modify and fine-tune the LLM with their specific data, like product details and customer information, thus enhancing task performance without compromising data security.
However, the decision to use open-source LLMs involves several considerations, including:
Customization opportunities vs. legal considerations: The ability to tailor open-source LLMs to specific marketing objectives significantly enhances workflow and output. However, this customization comes with legal complexities, especially concerning copyright issues related to training data. Unlike Google, OpenAI, and Microsoft, which provide indemnification against copyright claims, the responsibility with open-source LLMs falls to the user.
Internal data access vs. security risks: Using open-source LLMs enables IT departments to host and process data internally, improving security and compliance. This setup allows marketers to access sensitive company information in their queries to create more relevant content and conduct deeper data analysis. Nonetheless, it transfers the responsibility for data privacy, governance, and compliance to IT teams.
Cost-efficiency vs. implementation complexity: While open-source models are free, like a puppy is free, their deployment requires significant technical know-how and resources, which may negate some cost savings. Collaboration between marketing and technical teams is crucial to evaluate feasibility and maximize the models' effectiveness.
Choosing the right LLM for your organization
Despite the buzz around open-source models, the ease of use and integration offered by commercial LLMs often make them the better choice for most marketing departments. Solutions like ChatGPT Teams and ChatGPT Enterprise, which exclude data and conversations from training their models, and Google’s Gemini in Workspace and Microsoft’s Copilot in Office, which integrate LLMs directly into productivity apps, typically offer greater value for most organizations.
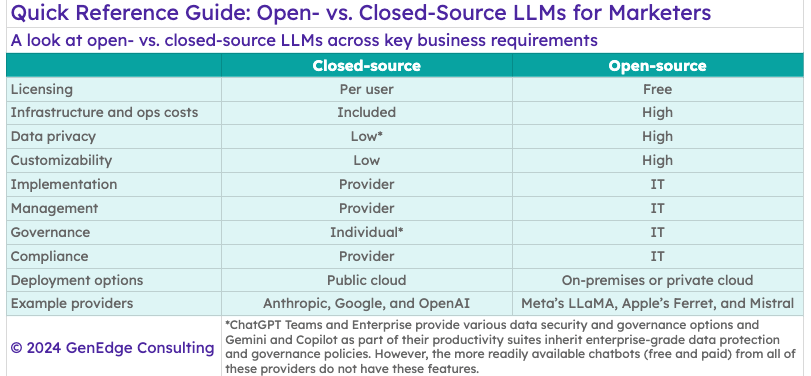
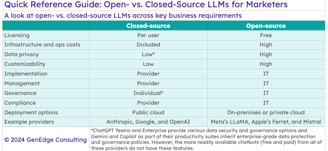
However, open-source LLMs may be suitable if your organization currently prohibits commercial models due to security concerns, or if you want to build an internal marketing app that requires access to sensitive data, such as one that gives marketers access and the ability to analyze website traffic, campaign metrics, or customer information. By managing these solutions within a secure, internal environment, IT teams can mitigate many security risks associated with external data processing and the training that occurs with commercial solutions.
While open-source LLMs offer intriguing possibilities for marketing teams, particularly in terms of model customization and use of internal data, they come with their own set of challenges and responsibilities. Ultimately, choosing to adopt an open-source LLM requires a balanced assessment of your organization’s capabilities, needs, and legal constraints and should be a collaborative decision with your legal and IT teams to fully understand data usage, intellectual property, and implementation implications.
AI disclosure: This post was AI-enhanced with Perplexity and ChatGPT based on a short opinion piece I wrote to a reporter. This post’s image was AI-directed with DALL-E. I, Natalie Lambert, contributed as the human element in these activities.